The cutting edge in data ethics
Ethics has been a top priority at Fable Data since day one. Handling consumer data, even anonymised and at an aggregate level, brings with it a significant responsibility. Our Data Science Team actively checks for sensitive features in the data used to develop and support Fable’s products, which includes maintaining a whitelist of acceptable features and iteratively checking for bias in both the data and the models it helps develop.
Fable is not certainly not alone in shouldering this responsibility. Globally, data-intensive companies are facing increased calls for transparency and accountability. Recent developments include, for example, the General Data Protection Regulation’s provisions around data protection impact assessments, or Europe’s High Level Expert Group on Artificial Intelligence’s guidelines and checklist for ethical and trustworthy AI. Couched in the language of data ethics, consumers and society are increasingly demanding AI products and services which are not simply more accurate and reliable, but also trustworthy.
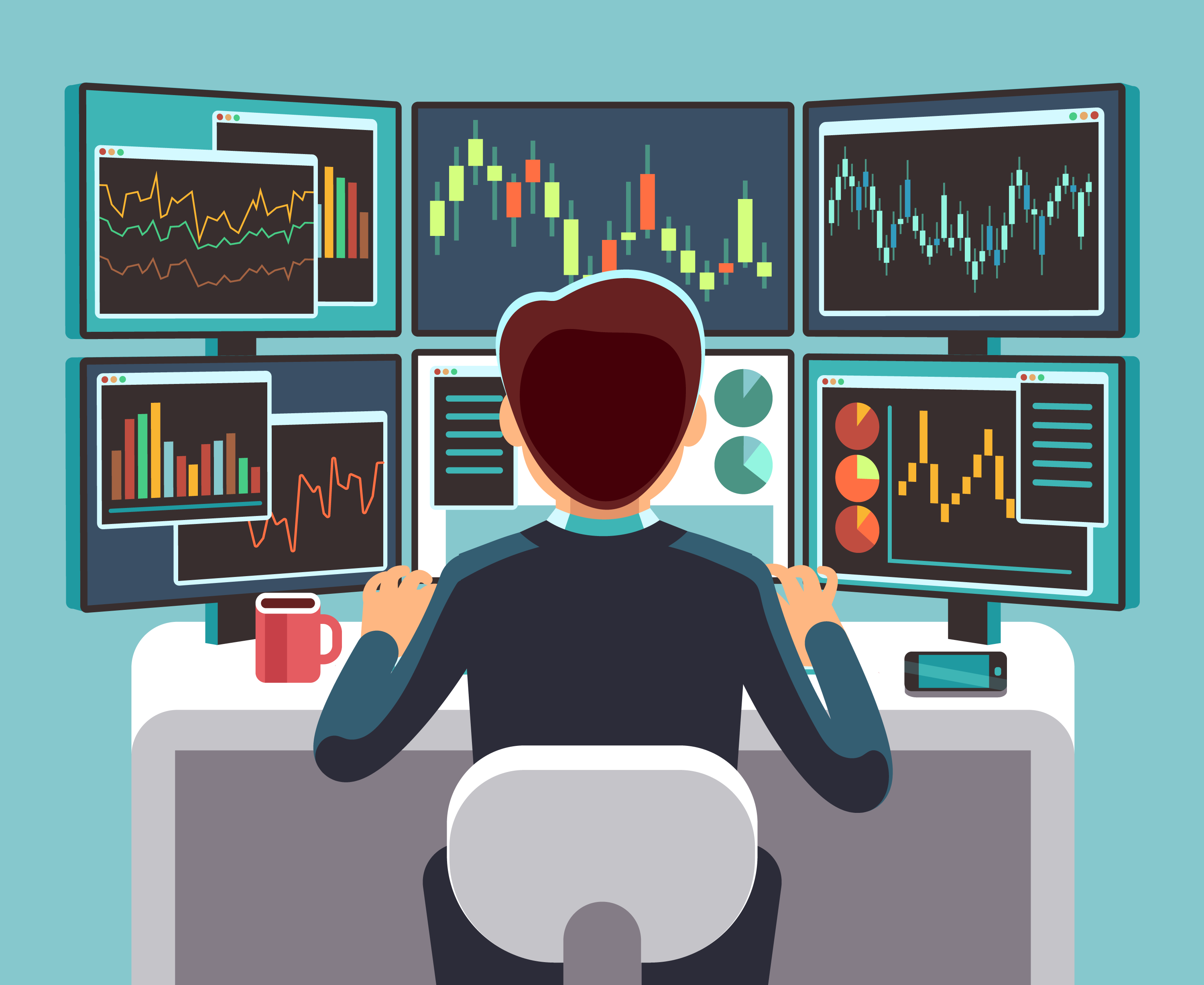
This greater expectation for public accountability is entirely reasonable. Machine learning understands the world through data, which inevitably reflects the biases, presumptions, and blind spots of people who create training sets, select features and tune parameters. Consumer data reflects reality and society as it exists, both for good and bad. If we are not careful, machine learning models will implicitly learn, replicate, and hide our biases and gaps in representation in a ‘black box’, entrenching and spreading problematic prejudices and beliefs about the world into models intended to help us make more objective and accurate decisions. Many of the most difficult ethical questions facing AI can be traced back to the provenance of the data used to train machine learning models.
To support this critical work to develop ethical products and models, it is essential to stay on top of the latest developments in research and policy-making concerning ethical and trustworthy machine learning and data-driven products and services. Researchers, major technology companies, and civil society are now driving forward the development of various tools, methods, and standards to detect bias in models and datasets, and to provide essential information to regulators, consumers, and the general public.
These tools can help clarify the provenance of datasets and models, their intended usage, and contexts or tasks for which they are well-suited or a poor choice. Many of these initiatives focus in particular on identifying potential biases, gaps, proxies for sensitive variables (e.g. ethnicity), and correlations which could be inadvertently picked up and reinforced by machine learning systems making use of the data. This information can help customers, policy-makers, researchers and the general public to understand what and how data is being used, and the extent of potential biases.
Across the field several types of tools have been developed. For datasets, standardised forms of documentation can be used to explain how the dataset was created and composed (e.g. variables, data sources), including how the data was collected, cleaned, and distributed, known ethical and legal considerations, as well as statistical tests commonly performed prior to deployment. For example, ‘datasheets for datasets’ are documents meant to be filled out by machine learning developers and attached to the datasets used to train their models. A similar approach, the ‘dataset nutrition label’, includes not only standardised documentation but a modular set of tools designed to minimise the time between data acquisition, model development, and deployment. These types of documentation can provide essential information and technical features necessary for the ‘exploratory’ phase immediately following data collection.
Comparable initiatives exist for trained machine learning models themselves. ‘Model cards for model reporting’are a short set of documentation meant to accompany trained machine learning models that describes various performance characteristics and intended uses of the model. The cards evaluate how performance varies by context when applied to datasets representing different cultural, demographic, phenotypic and intersectional groups. Similarly, a ‘standardised declaration of conformity’ for AI has been proposed to provide consumers with crucial information concerning the purpose, performance, safety, security, and provenance of AI products. Consumer-facing tools are particularly imported to ensure data-intensive products remain accountable and trustworthy in the eyes of consumers.
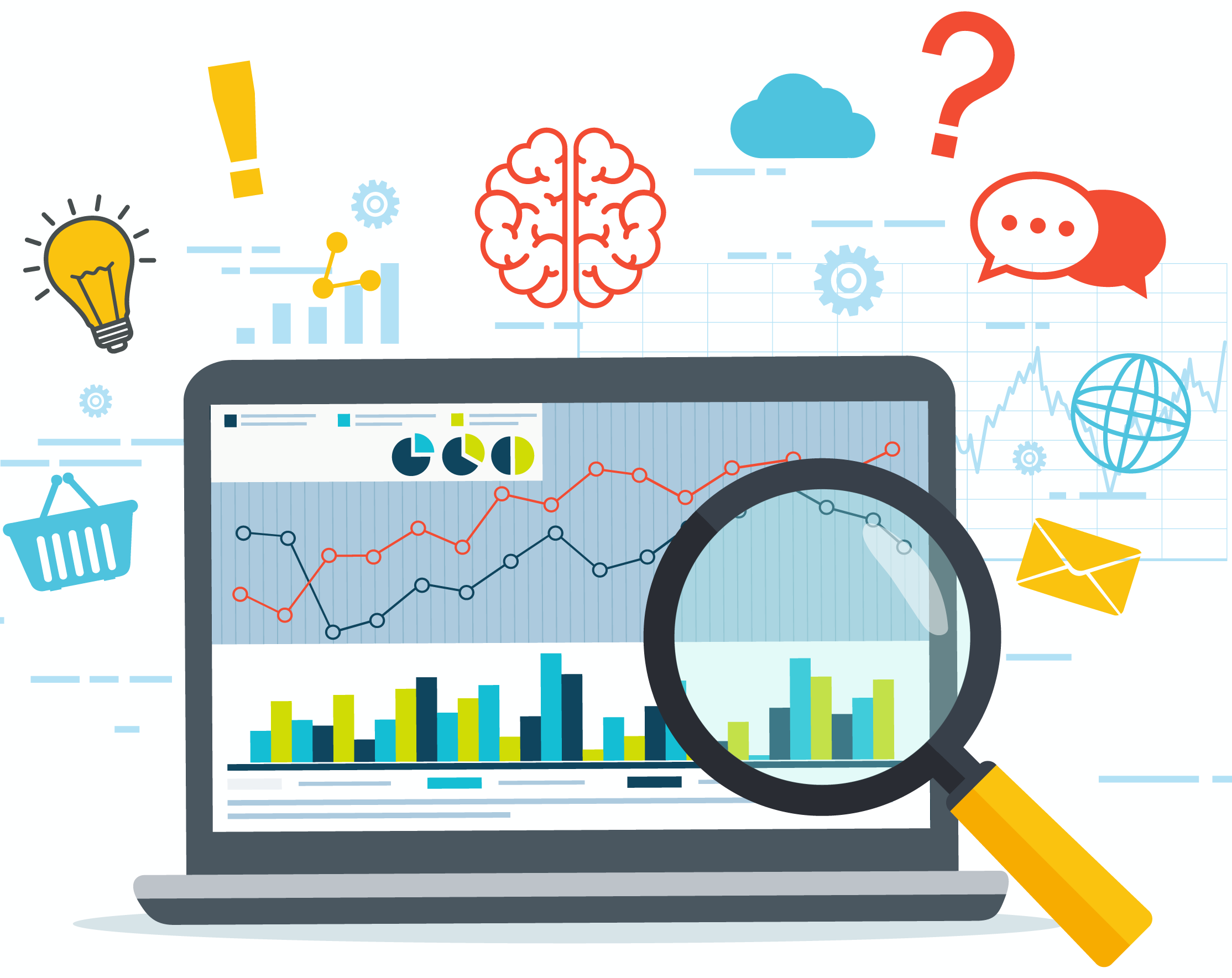
This type of standardisation is certainly not unprecedented or limited to data-driven products and services. Documentation standards have been voluntarily adopted in many other industries to consistently describe the provenance, safety and testing carried out on products prior to release.
Numerous toolkits have also been developed to assess and correct for bias and fairness in datasets and models at any stage of commercial deployment. The AI Fairness 360 toolkit, for example, includes numerous tests and algorithms to measure fairness and mitigate bias in datasets and models. The toolkit aims to help bridge the gap between research on bias and fairness in AI and real-world AI products and services. Similar initiatives can also be found, such as the Aequitas project which has developed an open-source toolkit to audit machine learning models used in predictive risk-assessment for discrimination and bias.
Several tools have also been developed to support the developers and data scientists on the front line of tackling bias. The High Level Expert Group on Artificial Intelligence, for example, has proposed a wide-ranging assessment checklist to support companies in developing ethical and trustworthy AI. Similarly, the IEEE Global Initiatives on Ethics of Autonomous and Intelligent Systems is developing a series of industrial standards and curriculum to support ‘Ethically Aligned Design’in machine learning and AI. Most recently, researchers have co-developed a ‘fairness checklist’ to help development teams talk about bias and fairness in a structured way.
Commercial, political, and social interests align in the documentation of provenance and testing for bias in datasets and models. Documenting datasets and models is a low-cost, high-yield first step towards responsible machine learning and data-driven products and services. These toolkits provide precisely the type of information and evidence needed to ensure datasets and trained models are being used as intended and with awareness of their limitations. Firms that seriously engage with their ethical responsibilities and commit to developing more transparent and less biased products will increasingly see a competitive advantage in a marketplace that demands accountability. Adopting these tools is thus in the interest of all parties involved. Tackling the problems of bias and fairness is both a moral and legal imperative, and increasingly a competitive advantage.
It is considerations such as these that has driven Fable Data to embed ethics from the very start, and to take seriously its responsibility to detect and avoid potentially biased and sensitive data and features in its products. These sorts of tools to assess the provenance of data, and to detect and correct for biases in datasets and trained models, will be a key tool to ensure ethical and trustworthy AI and data-driven products and services in the future.
Brent Mittelstadt
Dr Brent Mittelstadt is a Research Fellow and British Academy Postdoctoral Fellow in data ethics at the Oxford Internet Institute, a Turing Fellow and member of the Data Ethics Group at the Alan Turing Institute, and a member of the UK National Statistician’s Data Ethics Advisory Committee. He is an ethicist focusing on auditing, interpretability, and ethical governance of complex algorithmic systems. His research concerns primarily digital ethics in relation to algorithms, machine learning, artificial intelligence, predictive analytics, Big Data, and medical expert systems.