The Great Alternative Data Hack
Fable came in third out of twelve teams in the first European Alternative Data Hackathon, organised by DataScrum in collaboration with a number of alternative data providers and hosted at the Microsoft Reactor in Shoreditch.
DataScrum is a community of data scientists and data science enthusiasts based in London. They organize events in the alternative data space focusing on finance and have a growing community on meetup. Their past events have included speakers from Jupiter Asset Management, Citadel, Janus Henderson, M Science, Refinitiv and more. DataScrum’s aim is to build awareness and educate people on alternative data in the tech community here in London which has been lagging up until now. They are also a talent discovery platform and source the right candidates for their partners and clients to recruit, offering a bespoke talent discovery service to fill in the growing demand for data scientists in the alternative data space. If you’ve missed the Alternative Data Hackathon or just want to hone your data science skills and work with actual datasets, you can join their workshop, the first Alternative Data and Data Science for Finance workshop in London on 16th November.
“The Great Hack — Inaugural London Alternative Data Hackathon in Finance”… First of its kind, the Hackathon was designed to bring together many bright minds in the world of finance, practitioners and enthusiasts alike, to demonstrate the power of alternative data by working on one or more of the six problem statements identified by Datascrum. The teams combined data from multiple sources to build investment strategies. The sources of alternative data included web traffic and app usage data from Similarweb, high frequency financial estimates from Refinitiv, brand frequency data from M-Science and location data from Location Sciences. We chose to address the problem of being able to build a high frequency (daily data/daily trading signals) investment strategy using multiple sources of alternative data.
Typically, the management of a business guides the community of analysts covering a stock either by explicit guidance from time to time or by offering insights into the business, and at times even making data publicly available via the investor relations arm. The analyst community re-rate their expectations and publish new guidance to investors, who in turn adjust exposure to the stock or enter new positions to reflect this new information.
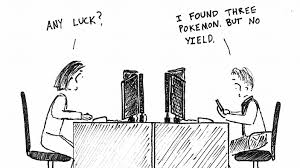
What if investors could tap into a source of information similar to that of the management peering into the coffers of a corporation to determine in real time the state of health of the business? We could predict prices and follow momentum on the predictions to invest in the stock accordingly. My colleague, Jonathan Franco, will publish details about our strategy and the data science techniques employed to develop the strategy.
(α)‘Alt-pha’ in a (β) world…
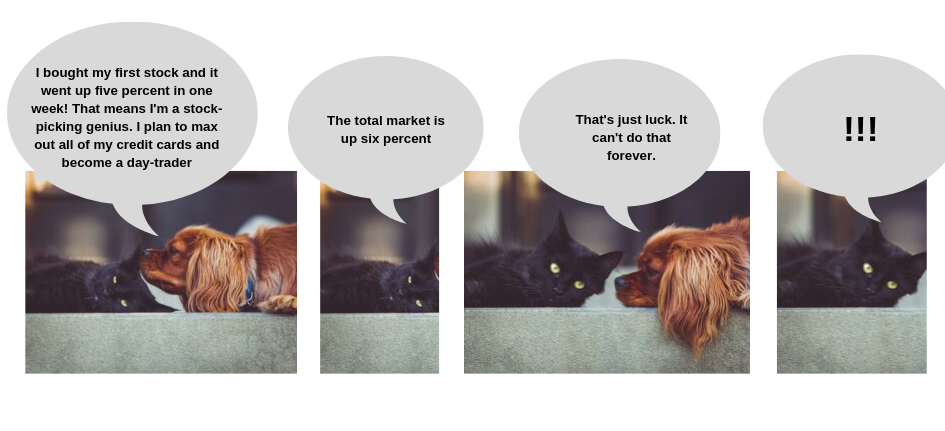
“We continue to make more money when snoring than when active.” Warren Buffet
Data driven investing has been on the rise through much of this decade with professionals constantly on the look out for the next treasure trove of alpha. Alpha comes from many sources, much of which used to be a managers ability to pick idiosyncratic investment opportunities , disciplined approach to managing risk and most importantly the manager’s prowess to time the market. Market timing is often driven by an informational advantage related to the market microstructure, a deep understanding of investment flows that drive significant price action and the ability to stay a few steps ahead of the herd by being able to source and process complex but publicly available information about the underlying business.
As the father of modern investing, Benjamin Graham, put it… “In the financial markets, hindsight is forever 20/20, but foresight is legally blind. And thus, for most investors, market timing is a practical and emotional impossibility.”
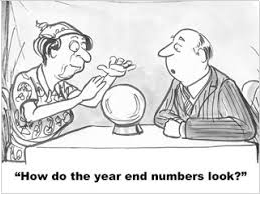
Many of the sources of alpha mentioned above have become harder to source as markets constantly change, information flow becomes more obscure, liquidity characteristics change and the rise of beta scalping (passive or smart) has increasingly dominated price action. As traditional sources of alpha diminish, managers are employing armies of quant’s and data scientists in the crusade to find new sources of alpha on the distant shores of the alternative data universe. We know the predictive power of alternative data as a leading indicator of stock price movements, so it is not inconceivable that alpha from “timing the market” can be recreated.
We were up against some very formidable opponents, all of whom brought along very interesting perspectives on one or more of the six targets. We chose to go after the high frequency (daily trading) using web, app and brand data. Some team focused on predicting earnings ahead of reporting periods and trade an earnings beat or miss versus analyst expectations while the rest attempted to solve either a macro use case or a use case involving location data or gaming data.
The teams had five data sources to scour for alpha:
- Similarweb: website usage from desktop and mobiles, mobile app usage data, offering information about activity such as users active, time spent online or on the app, bounce rates for web pages, penetration rates as % of the overall panels etc.
- Refinitiv: price and volume data, but more importantly offered consensus estimates for Revenue, Earnings Per Share, Net Margins etc
- Location services: foot fall data in high density regions in London, along with information about distances travelled to and from the location. Helpful when predicting REITS in the context of the Hackathon
- Mscience: brand frequency data that offers insights into how a brand is perceived on social media
- Gaming Data: Steam API and Twitch for gaming data (used for gaming stock such as electronic arts)
The winning team presented compelling evidence of the use of web traffic and mobile app usage data as leading indicators in predicting earnings ahead of announcement periods. They employed bottoms up approach by narrowing down the list of stocks to those that exhibited good correlation between announced earnings and the leading indicators. In order to do, they adjusted/normalised many attributes of the panel to reflect a more realistic estimate of user activity and then eliminated biases to changes in the panel (such as large swathes of new panel participants being added). Armed with a normalised panel, they employed standard techniques and regression analysis to arrive at a predictive model that allowed a user to position for earnings a good fortnight ahead of the event and to trade the stock accordingly.
The team that was placed second brought along a Statistical Arbitrage strategy. While stat arb has become a fairly common strategy, there exist many variations and beta sizing of the stock pair is a key factor to superior returns. The team demonstrated that the alternative data sources used enhanced the results of their strategy. When relationships between the alternative data related to the pair of stocks being traded changed significantly, the model resized trades to a more appropriate beta or exited active trades ahead of big price swings that lead to disruption in pair wise correlation.
My personal favourite was team Litqudity (with Applied Data sciences). They chose to use gaming data from alternative data providers in the gaming space to predict the earnings or stock price movements of gaming providers like Electronic Arts. The team approached the problem by looking at the financial statements of the business to understand geographic revenue splits, geographic biases to certain gaming apps developed by the business and sector level data from gaming sector database. Using this information they narrowed their analysis and efforts down to a single stock that held the most promise. The team created features from the various alternative sources but was left with little time to complete the exercise. Using well over 6 data sources, aggregating and scrubbing all of this data and then quantifying fundamental data not available in any of the other sources, I have no doubt the approach would have yielded some very good results or very interesting observations when modelling revenue for a geographically diverse brand.
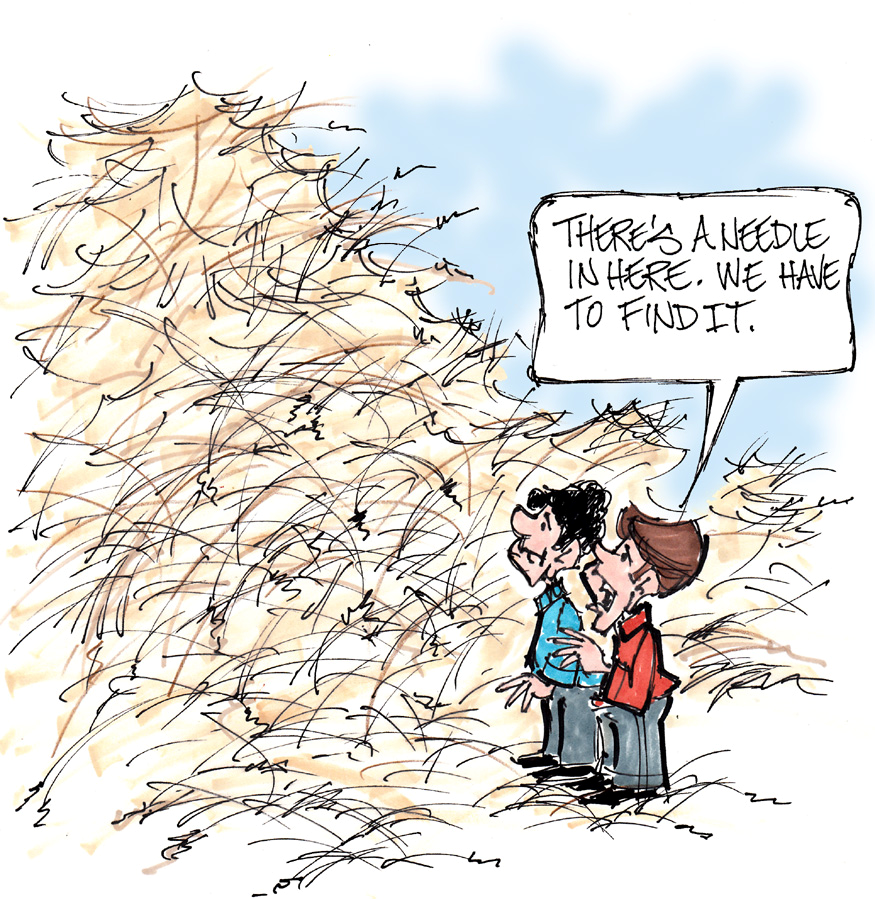
- Alternative data has value and can definitely offer alpha from a timing perspective.
- In all instances, winning teams demonstrated that information in the alt data source were leading indicators.
- Panel volatility and stability was a key stumbling block. Being able to normalize the panel was one of the key parameters in the success of winning teams.
- Finding the right feature/KPI can be akin to finding a needle in a haystack. Starting with a fundamental premise can be very helpful in framing the problem and can drive successful identification of relevant KPI’s